1 Blast from the Past Lesson if You’re Interested in AI
![]() |
By Jurica Dujmovic |
From transforming business operations to reshaping consumer interactions, AI’s influence has permeated every corner of modern life.
Yet, beneath this veneer of unstoppable advancement, a crucial question lingers in the minds of investors, technologists and economists alike: Are we witnessing the formation of an AI bubble akin to the notorious Dot-Com Bubble of the late 1990s?
Answer: We may be.
Let me explain …
As the AI industry's market value skyrockets, experts are drawing parallels.
They note a similar pattern of exuberant investment and lofty expectations. Venture capitalists are pouring vast sums into AI startups. Many of which have yet to demonstrate profitability or even viable products.
Much like their Dot-Com predecessors.
Yet, AI is not a fledgling field. It has deep roots in decades of research and development. Its applications are not just theoretical but have shown practical, transformative impacts across various sectors.
This juxtaposition of AI’s tangible successes against the backdrop of speculative investments forms the crux of the debate. After all, how can a bubble burst if the technology underpinning it is solid and useful? Could the current AI struggles just be the growing pains of a technology that is genuinely reshaping the future?
Let’s start by looking closer at the Dot-Com Bubble.
The concept of a bubble isn't new. It's a recurring phenomenon in the history of economic cycles and is particularly noticeable in the technology sector.
And the Dot-Com Bubble was characterized by a frenzied rush into internet-based companies. Hype was so high that investment in dot com firms reached a fever pitch, fueled by the widespread belief that the internet would revolutionize business and consumer behavior.
And it did. The internet is arguably one of the most influential technological advancements in history.
So, what happened? Why did most Dot-Com investments go under when the bubble burst?
Because they weren’t designed to last.
Startups with little more than a “.com” in their name attracted massive investments regardless of the quality of the underlying business. This led to sky-high valuations without the backing of sound business models or revenue streams.
Many had no clear path to profitability. But they were able to collect investor dollars just by virtue of being an internet play.
So, when the internet’s growing pains did become evident, when the expectations driven by hype couldn’t be fulfilled immediately, the bubble burst. And it took out all those companies that hopped into the trend without a long-term plan, and then some.
And wiped out trillions of dollars in market value in the process.
We’re starting to see a similar trend with AI. Harvard Business Review wrote in June 2023 about how AI is distracting companies. The argument is that the term is so broad, it encompasses all that machine learning has to offer now and in the future.
And because of that interpretation, we’re seeing an unprecedented surge in both the adoption of AI technologies and investment inflows. All in a relatively short time.
A recent survey by McKinsey says that by mid-2023, 79% of people polled have had at least some exposure to generative AI — like ChatGPT — both in and out of their work. Of those, 22% are regularly using it in their own work.
According to Statista, the AI market will grow at a compound annual growth rate of 17.3%, from $242 billion in 2023 to $585 billion by 2028.
At that rate, that means experts anticipate the AI sector will double in size every year until then.
AI Market Size by Year
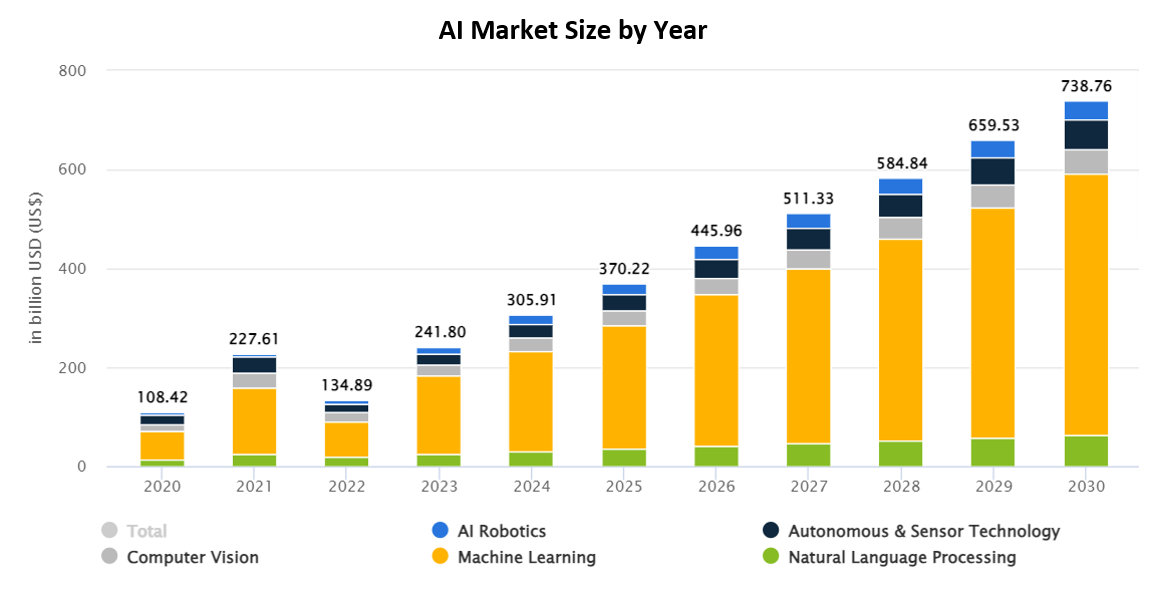
Indeed, a significant number of organizations are already integrating generative AI regularly in their business functions.
We’re officially in a new era in AI applications.
And the money is following the adoption. AI high performers — companies at which AI contributes significantly to their earnings — are channeling over five times their digital budgets into AI. This is a clear indicator of the strong belief in the return on investment AI offers.
But as I mentioned earlier, there are limitations to AI that the hype isn’t covering. In fact, it’s straight up ignoring in many instances.
Media hype and investor sentiment can play a significant role in inflating bubbles. During the Dot-Com Era, relentless media coverage and the fear of missing out drove investors to pour money into internet stocks without due diligence.
A similar pattern can be observed in the AI sector. The sensational coverage of “artificial intelligence” breakthroughs might be contributing to inflated expectations.
That’s because, in reality, there is no “intelligence” at all in current AI offerings. And while innovation is a key driver of economic growth, distinguishing it from irrational exuberance is essential when it comes to deciding what to do with your investment dollars.
AI at its core is all about pattern recognition. It can respond to a prompt by “predicting” what will come next … based on data references of what has come before.
The more data a platform can pull from, the better it can craft a more accurate response. But without good or sufficient data to pull from, AI platforms can shoot out pure nonsense. This means that, while the promise of AI is undeniable, its current limitations and challenges often contrast starkly with the optimistic portrayals in media and investor circles.
Actually, there are four main ways that current AI tech falls short of popular hype:
- Lack of accuracy: Also called AI hallucination, this is one of the more peculiar yet significant shortcomings of AI. When a program’s data set is limited in any way, AI has a tendency to "hallucinate" or generate misleading, incorrect or nonsensical information.
This phenomenon is particularly prevalent in complex AI models like large language and image generators, which can produce convincing but entirely fabricated content. This not only questions the reliability of AI-generated information but also highlights the challenges in using AI for critical decision-making processes.
- Dependency on quality datasets: As mentioned above, AI's effectiveness is heavily reliant on the quality of the datasets used for training. In many cases, datasets are incomplete, biased or unrepresentative of real-world scenarios. This leads to AI models that are inaccurate or biased, significantly limiting its functionality and usefulness. Especially for sensitive applications such as decision-making, recruitment and law enforcement.
- Vulnerability to data exploitation: AI systems can be manipulated or "tricked" into divulging sensitive or confidential information, making them a potential security risk. While most models have parameters guiding the AI’s responses, skillful prompting and exploitation of a model’s weakness can get around those guidelines. Thus, information that should have remained confidential is leaked.
This vulnerability is a serious concern, particularly in the context of data privacy and security.
- Need for human oversight: The current state of AI technology does not allow for full automation without human intervention or oversight. AI systems often require human judgment to assess and correct their output, especially in complex or nuanced tasks.
This limitation is particularly evident in fields like medical diagnostics, content moderation and legal analysis, where the subtleties of human experience and ethical considerations are paramount.
All this means is that while the impact of AI is being felt across various industries — including IT, telecommunications, healthcare, BFSI, automotive and retail — the technology is nowhere near replacing the people in those roles. It’s still just a tool.
So, headlines about AI being able to do exactly what a human can … are just adding to the hype.
No matter how the AI sector progresses from here, there is one lesson you should take away from the Dot-Com Bubble: Be wary of speculative investments that are disconnected from the current fundamental value of the underlying asset or technology.
In the case of the Dot-Com Bubble, many companies were overvalued based on potential future earnings rather than current performance.
This is a cautionary tale for the AI sector, where the potential of AI is immense, but the actual value realization might still be in its infancy.
But, as I said, none of these shortcomings undercuts the value inherent in this new tech. It simply means investors need to judge an opportunity based on what it can deliver now … not the potential promise of tomorrow.
That leaves me with just one question — one I hope you consider as well: How can we tell if we are in an AI bubble?
For my answer to that, check your Weiss Crypto Daily next Thursday.
And in the meantime, please write to us on X by tagging @weisscrypto to let me know what you think is in store for the future of AI!
Best,
Jurica