Bigger Opportunities Will Come in Smaller AI Packages
![]() |
By Jurica Dujmovic |
I’ve talked previously about the intersection between artificial intelligence and the crypto space. AI-generated NFTs were a main narrative in the sector in 2023, and as AI continues to develop, the ways in which it can be applied to crypto projects will expand.
That’s why today, I’m taking a step back to review exactly what is going on in AI development, which has lately been nothing short of revolutionary.
Indeed, machines are now capable of learning and performing tasks that were once considered the exclusive domain of human intellect.
The current state of artificial intelligence is marked by groundbreaking advancements, encompassing sophisticated language models like OpenAI's GPT-4 and Anthropic’s Claude, as well as the multitude of open-source intelligent algorithms popping up in the thousands daily.
However, these achievements used to come with a significant caveat: the immense computational power required to train and run the majority of these gargantuan models.
A study by OpenAI in 2018 revealed a startling increase in the computational power used to train the largest AI models. Since 2012, the amount of power needed had doubled every 3.4 months.
That’s seven times faster than the historical trend observed over 50 years from 1959 to 2012!
This exponential increase is exemplified by the fact that computer usage for such models surged by 300,000-fold in just the past seven years.
This trend not only signifies the escalating costs associated with the field’s achievements … but also raises concerns about sustainability and scaling, since these increasing computational costs directly translate into higher maintenance and development costs.
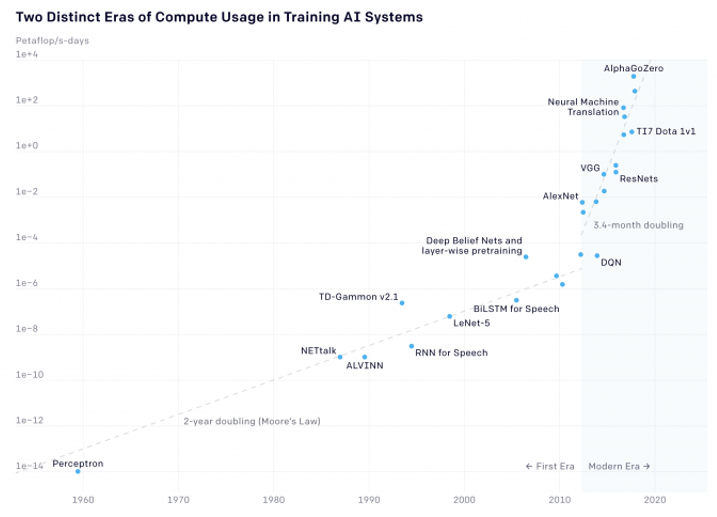
This rapidly growing demand for computational resources has led to questions about the viability of mass AI adoption.
The main concern is that the high processing requirements might create a bottleneck, hindering the widespread deployment of AI technologies.
This may have been true in the past, but if recent development in the industry is any indication, the reality is quite different:
Indeed, recent advancements in AI technology suggest that the computational power needed to train new models is not necessarily increasing.
Three factors contribute to this trend, making AI development more efficient and accessible:
Factor 1: Optimization and Sophistication of AI Models
New AI models are becoming optimized and sophisticated, capable of achieving more with less computational complexity. Lincoln Laboratory, for instance, is actively working on methods to reduce power consumption and train AI models more efficiently, making energy use transparent in the process.
Moreover, techniques are being developed to predict the performance of AI models early in the training process, enabling underperforming models to be stopped, leading to an 80% reduction in energy used for model training.
Factor 2: Development of Energy-Efficient AI Chips
The design of AI-compatible chips is rapidly advancing, significantly increasing AI capabilities without excessive power expenditure.
An example is the AI-ready architecture developed by Professor Hussam Amrouch at the Technical University of Munich. These chips use ferroelectric field effect transistors (FeFETs) and are designed to be twice as powerful as comparable in-memory computing approaches, with 885 TOPS/W (tera-operations per second per watt) efficiency, which is a key measure for future AI chips.
Factor 3: Growth of Open-Source AI Models
The open-source community is playing a crucial role in AI development, providing a multitude of models for free on platforms like GitHub.
In 2023, there was a notable increase in developers building with generative AI, and open-source generative AI projects even entered the top 10 most popular open-source projects by contributor count.
This surge in open-source AI innovation is driven by individual developers and marked by a 248% year-over-year increase in the total number of generative AI projects. Among these models, small and agile 2-13 billion parameter language models (SLMs) are becoming increasingly more important.
These models operate at substantially lower costs and are particularly suitable for environments where computational resources are limited. Their streamlined architectures enable rapid processing and quick decision-making, making them highly suitable for real-time applications.
This efficiency and responsiveness position them as strong competitors in various domains.
Here are just a few of the most notable ones currently giving their larger counterparts a run for their money:
- Microsoft's Phi-2: Part of Microsoft's suite of smaller, more nimble AI models, Phi-2 is a 2.7 billion-parameter language model. Despite its relatively smaller size, Phi-2 can outperform large language models — or LLMs — up to 25 times larger, showcasing that smaller models can indeed compete effectively with larger models in terms of performance.
- LLaMA-2: A compact yet powerful AI model with 7-70b parameter versions available, LLaMA-2 is designed to match the capabilities of larger models like GPT-4. LLaMA-2 excels in various language tasks from text generation to question answering, showcasing that smaller models can achieve high performance with reduced computational demands. Its efficiency and adaptability underscore the growing significance of smaller models in advanced AI applications.
- Microsoft's Orca AI: This 13-billion parameter small AI model is designed to imitate the complex reasoning process of large foundation models like GPT-4. Orca AI leverages rich signals, including explanation traces and step-by-step thought processes, to bridge the gap between small models and their larger counterparts. It has demonstrated impressive performance on complex reasoning benchmarks, emphasizing the potential of smaller models in high-level reasoning tasks.
- Mistral 7B and Zephyr 7B: Developed by Mistral AI, Mistral 7B is specifically tuned for instructional tasks and has been shown to be efficient, delivering similar or enhanced capabilities compared to larger models with less computational demand. Building on this, Hugging Face's Zephyr 7B, a fine-tuned version of Mistral 7B, surpasses the abilities of significantly larger chat models and even rivals GPT-4 in some tasks.
Overall, the smaller size of these open-source models has six key benefits:
1. The smaller size leads to faster training and quicker development and iteration cycles. This agility is crucial in a rapidly evolving field like AI, where frequent updates and improvements are necessary to stay relevant.
2. Smaller models are also more energy-efficient, as noted earlier. This not only aligns with sustainability goals but also reduces operational costs. And that is particularly important for startups and smaller companies that might not have the resources to invest in the hardware required for larger models.
3. Due to their manageable size, smaller open-source models can be fine-tuned more easily for specific tasks or industries. This flexibility enables businesses and researchers to tailor models to their unique requirements without the need for extensive computational resources.
4. Smaller open-source models can process data and provide outputs more quickly, which is crucial for applications that require real-time responses such as mobile apps, interactive tools and edge computing devices.
5. While large models often require vast amounts of data to train effectively, smaller open-source models can achieve good performance with considerably less data. This aspect is particularly beneficial when dealing with niche domains or languages where large datasets may not be available.
It also makes these models more accessible to a wider range of users and developers, including those in academia, small businesses and developing countries. This democratization of AI tools fosters innovation and broadens the scope of AI research and application.
6. Last but not least, these smaller open-source models can be more easily deployed on-device, reducing the need to transmit sensitive data to the cloud. This on-device processing enhances privacy and security, making these models preferable for applications handling sensitive information.
The underlying thread is clear:AI is not just about bigger data and more power-hungry models; it's about smarter, leaner computations that democratize access and empower innovation on a global scale.
This shift toward using less to do more is not only economically sound but also socially responsible, ensuring that AI is not just a privilege of the few, but a benefit of many.
As it proliferates and becomes more accessible, it’s bound to be utilized even further by various cryptocurrencies and dApps within the blockchain to the benefit of both — including smaller projects with less capital.
Which cryptocurrencies dApps are most likely to be pioneers in bridging AI and blockchain technology is a question I will answer in an upcoming issue.
Until then,
Stay safe and trade well,
Jurica